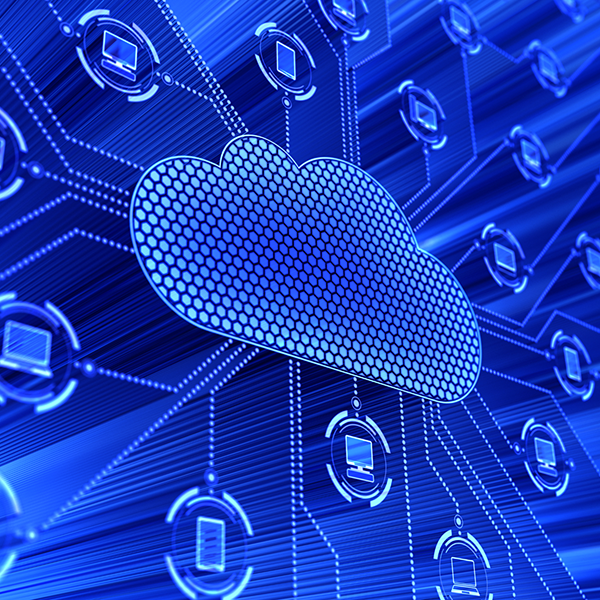
Blog | 9/29/2021
AI in Healthcare: Insights from Two Decades of FDA Approvals
Artificial intelligence (AI) may be the single most hyped (some would say overhyped) innovations in healthcare of the last 20 years, with countless, often breathless promises of everything from modest efficiency gains to a complete transformation of healthcare.
This is especially true for the subset of AI known as machine learning (ML), a set of techniques for using data and algorithms to imitate the way that humans learn, identifying patterns and meaning in a human-like way, but with much greater efficiency and insight, and with the tantalizing potential for the algorithm to “learn” and improve over time.
While impact to date has not yet lived up to the grandest fantasies of AI/ML proponents, there has been meaningful progress across many areas, including:
- Healthcare provider process automation
- Drug discovery
- Medical imaging analytics, especially in radiology but also in pathology, ophthalmology, and dermatology
- Robotic surgery
- Disease screening and diagnosis from sensor data, especially in cardiac disease
- Disease management, particularly in diabetes using CGM data
- …and many others
Most of these innovations have not gone through review by the FDA or international regulatory bodies, and those that have are often supported only by limited evidence.
Still, a careful look at what kinds of AI/ML tools (or “medical devices” in the language of the FDA) the FDA has cleared can reveal some useful insights into where provider-facing or patient-facing AI/ML innovation is happening in healthcare and who is driving that innovation.
That type of analysis has long been difficult (but not impossible) due to the absence of a comprehensive FDA database of AI/ML devices cleared by the agency.
Fortunately, that changed on September 22, 2021, when the FDA published just such a database for the first time. (Incredibly, the FDA had to compile this data largely from publicly available resources, including a recent effort by STAT News to create such a database on their own, which raises some basic questions about record-keeping within the agency, but set that alarming point aside for now.)
Health Advances has taken a careful look through the contents of this database and has distilled a few insights that might be useful to innovators, investors, and others with an interest in AI/ML in healthcare.
Insights from FDA’s Database of AI/ML Approvals and Clearances
- Regulated AI/ML Activity Has Exploded in the Last 4 Years
While the hype around AI has been around for over a decade, 2018 marked the year when the floodgates really started to open at the FDA. In fact, over 80% of FDA clearances and approvals of AI/ML devices have occurred in just the last four years.
Innovators need time to acquire, scrub, and annotate datasets; build and train AI/ML models; and generate evidence of the accuracy and value of those models. By 2018, a large and growing number had progressed to the point of being ready for FDA submission, and activity has only accelerated since, though it’s worth noting that the pace appears to have slowed in 2021.
- Most of the Action is in Radiology
AI/ML is particularly adept at analyzing images. Images include an enormous amount of information, much of which is difficult or impossible for humans to detect, even if highly trained. And human interpretation of images is slow, especially compared to computers.
For those reasons (and, not insignificantly, because there is money to be made by enabling radiology clinics to process more cases per day), radiology has been the most active area for FDA cleared/approved AI/ML tools, by far.
- It’s All About the Images
The vast majority of cleared devices are based on imaging analytics of some kind. While this is obviously the case within radiology, it is notable that this point holds in other medical specialties too. Image analysis is not the only thing that AI/ML is good at, but for the reasons outlined above, it’s a natural fit for healthcare.
For those reasons, we should expect to see increased innovation in the coming years in medical specialties that rely on imaging but not radiology, specifically oncology (digital pathology scans) and ophthalmology (OCT and fundus scans).
Eventually, innovators will bring more devices to FDA that are based on other capabilities of AI/ML beyond image analysis, particularly natural language processing (NLP), which is the ability for computers to process human language, including the messy and technical language found in physician’s notes, pathology reports, and other high value but unstructured sources of health data.
- The Imaging Equipment Companies are Leading the Pack
It should come as no surprise that the global imaging equipment companies are at the top of the leaderboard. GE owns the top spot, with twenty-two algorithms cleared to date (reflecting their commitment to their AI platform called Edison). Siemens and Canon own second and third place respectively. Philips stands out as a laggard, with only 4 clearances to date.
The other two companies in the top 5, are prominent names within the burgeoning category of companies focused entirely on AI imaging tools. Aidoc just raised a $66M Series C round, and Zebra Medical Vision was recently acquired by medical imaging equipment company Nanox for $200M.
- The Honor of Being the Very First Company to Win FDA Clearance of an AI/ML Tool Goes to….
Compumedics, a medtech company focused on diagnostic devices.
Way back in 1997, Compumedics won approval for a ventilatory effort recorder (i.e., a breathing monitor) used in polysomnography (PSG), the sleep studies used to diagnose obstructive sleep apnea and other sleep disorders. Their system included software that automated interpretation of sensor data for sleep staging, respiratory scoring, and detection of waking and leg movement events. This software was based on a neural network model, a term that most people still didn’t know about twenty years after this clearance.
###