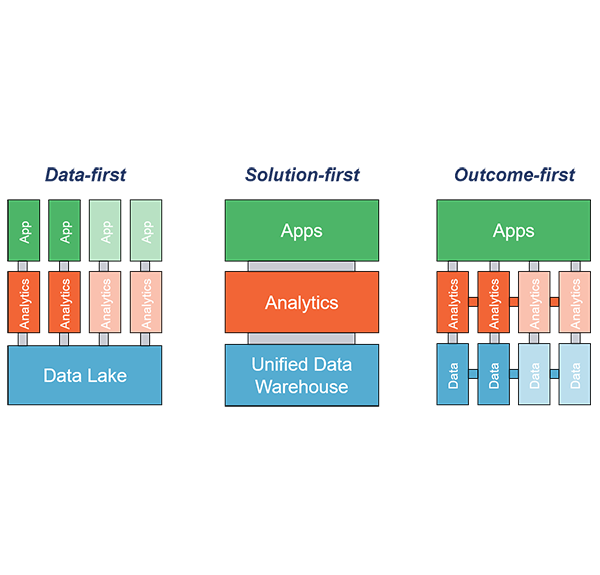
Blog | 4/4/2018
Three Models for Population Health Technology
By Greg Chittim, Vice President
As I reflect on the 2018 HIMSS conference and my many years of attending this event, I can vividly remember a conversation I had with a long-time industry veteran and analyst. After a long day of exploring the exhibit hall, he plopped down on a couch, put his face in his hands and said:
“I just spent 45 minutes talking to a sales guy who was convinced his company had the silver bullet for population health. It took me that long to wade through buzzwords and hand waving to figure out all they did was pop a new alert in a single EHR platform to remind a physician to do a screening.”
That was a refrain heard all too often in the early days of population health, when it was the buzzword of the year that no one could actually define. Depending on who you spoke with, it might have been really smart young consultants running around a hospital who didn’t know much about healthcare. Or a web application that hadn’t figured out how to interface with an EHR. Or a smartphone app that allowed (which really means required) physicians, nurses, and patients to change their workflow to communicate in real time. As we’ve all seen, solutions that don’t recognize the uniqueness of healthcare, are not interoperable, or won’t account for the inertia of workflow change management were all doomed to fail.
So have we made progress?
In the ensuing years, population health management solutions have generally agreed on a table stakes solution set similar to the following:
Where these solution providers differ is both the emphasis they place on specific use cases for a subset of their target users and the models by which they deliver solutions for those use cases. While there is still a wide variety of (and inconsistent evidence of) the most impactful use cases, the technology has solidified around three unique models, often defined by the company’s legacy as a solution provider, and their philosophy toward data, analytics, and applications.
- The Data-first model is centered around a Data Lake – gathering as much data as possible, in raw form, layering on top big data technologies to intuit connections, and building specific silos of analytics and applications for targeted big challenges like patient safety, measure improvement, and/or financial control.
- The Solution-first model focuses neither on gathering all the data, nor on iterative workflow approaches, but rather on a strong perspective on the single solution to managing risk and population health. This solution generally includes a simpler, unified dataset of EHR and claims data that requires upfront focus on data quality and sustainability. It also must present an interface that is simple enough for executive users setting strategy but with enough ad hoc analytics to make it useful to clinical analysts and office administrators who execute that strategy.
- On the other extreme, the Outcome-first model begins with the most pressing challenges in a health system or practice, determines what measurement or analysis is necessary to solve the problem, and then gathers only the EHR, claims, and ancillary data necessary to solve that specific problem. This process is repeated regularly against a prioritized list of challenges, which is updated regularly as national and local market forces and health system priorities evolve.
Which model will win?
There is no single model that will work for all hospitals, provider groups, and health plans needing to manage populations in a rapidly growing risk-based environment. That is why each of these models has a number of successful vendors delivering impactful solutions. The suitability of each model depends on the data landscape, the in-house analytical capabilities, and the approach to technology implementation present at each buyer.
Data-first Model
|
Summary: Gathers broad system-wide data with minimal transformation, supporting specific silos of analysis and presentation Common Legacy: Data warehouse technology providers Advantages: Robust departmental solutions with deep analysis requirements, often developed in conjunction with KOLs Disadvantages: Implementation time for the data lake and the related big data technologies will be long. Data quality can suffer as specific curation is difficult. Cross-department silos will continue to exist, as applications and analytics are rarely shared. Ideal Customer: Large IDNs that have access to a long history of claims data and just one enterprise EHR Prototypical Vendor: Health Catalyst (www.healthcatalyst.com) |
Solution-first Model
|
Summary: Aggregates broad clinical and cost data with strict transformation rules and a simpler dataset, supporting a single analytics engine and application platform Common Legacy: System integrators and data-centric consultants Advantages: Complete solution set for providers / payers in risk-based contracts. Offers a strong point-of-view on the tools needed to succeed. Disadvantages: One-size-fits-all approach harder to adapt. Focus on data quality upfront may frustrate buyers with “dirty” data. Ideal Customer: Health systems and provider groups with lots of EHRs and risk contracts that require harmonization Prototypical Vendor: Arcadia.io (www.arcadia.io) |
Outcome-first Model
|
Summary: Integrate specific clinical and claims data to drive specific, iterative outcome goals, revised regularly Common Legacy: In-practice consultants and quality improvement organizations Advantages: Faster implementation and rapid iterations. Ability to adapt to changing priorities at national, state, or local level. More immediate ROI. Disadvantages: Scaling to larger systems will be difficult without robust governance structure. Reliance on partnership with vendor for disciplines iteration. Ideal Customer: Midsize provider groups with a legacy of quality improvement and ability to adapt workflows on just a few risk contracts. Prototypical Vendor: Forward Health Group (www.forwardhealthgroup.com) |
Where should population health technology vendors go from here?
As we enter 2018, population health is a tech-enabled service supporting value-based care for healthcare providers, payers, patients that has matured immensely, and is finally ready for its graduation into adulthood. As an industry, population has moved beyond its infancy as an academic idea, grown up from the childhood of Meaningful Use, and matured through adolescence despite the frustrating lack of interoperability and usable data standards. As it enters a new phase of growth on the foundation of the three models discussed above, it will have to wrestle with some of the following challenges:
- Scalable integration and services – should integration be a core competency of population health vendors, or should they rely on those best of breed vendors like Datica, Redox, Ellkay, and Capsule?
- Social determinants of health – how do you layer in data beyond EHR, claims, and device data that may be just as or more important? Companies like Algorex are developing data-as-a-service to provide this important contextual data view into population health.
- Device data and active patient engagement – both in the hospital, on patients’ wrists, and on their smartphones, devices have an untapped set of real-time data that is rarely utilized by population health vendors. How might Apple HealthKit, Validic, Tactio, Capsule, Ascom Digistat, and the Fitbit/Twine merger drive a new set of insights into populations and individuals?
- Sophisticated risk stratification and AI-powered CDS – all population health vendors deliver some level of risk stratification, but how will AI, Natural Language Understanding, and clinical decision support allow for more regular and impactful patient engagement
- Telemedicine and home care integrations – texting appointment reminders and robocall telephone outreach are the core of today’s care management solutions, but click-to-call telemedicine functionality and supportive home care integrations will support faster and more effective resolution of care gaps
Population health technology will continue to be a turbulent and exciting area of healthcare to watch – stay tuned for deeper dives into some of the areas above!
About The Author Greg Chittim is a Vice President at Health Advances, a strategy consulting firm focused exclusively on commercial strategy and product development strategy for health technology innovators. He co-leads the firm’s Health IT and Digital Health practice and regularly works with health IT, medical device, and life sciences companies, as well as private equity investors on strategy in population health, patient engagement, interoperability, data monetization, and analytics. Follow Greg on Twitter: @chittim