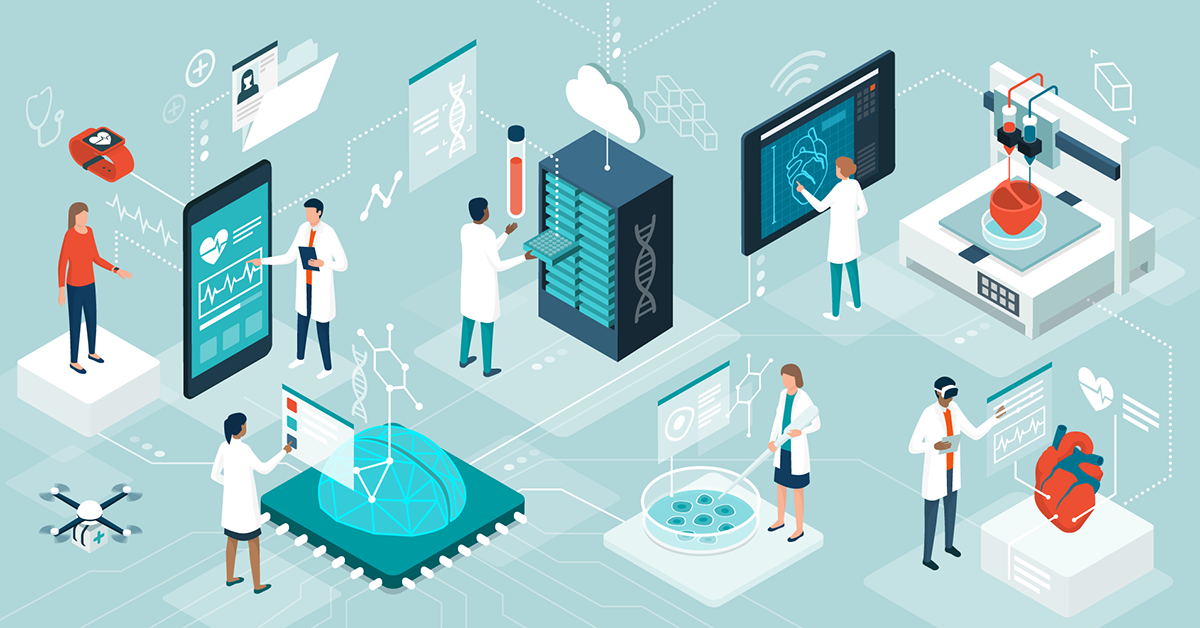
Blog | 3/8/2023
AI in Healthcare: Implications at the Molecular, System, and Patient Level
By Ashley Peake, with Contributions from Greg Chittim and Shomik Datta
This is the 2nd in a series of reports from the Wharton Healthcare Conference in February 2023. You can read the previous entry here: Is the Healthcare Industry Meeting the Rising Expectations of Consumers?
Introduction
Since its launch in November 2022, ChatGPT, the OpenAI and Microsoft-backed Large Language Generative AI model, has caused a lot of buzz about artificial intelligence technology. In 2023, ChatGPT passed the United States Medical Licensing Exam (USMLE). [1] This caused both excitement and concern for many, but more importantly opened the door for more central conversations about AI’s application in healthcare.
AI’s sizeable and growing market in healthcare has led to further interest from end-users, investors, and start-ups. GlobalData estimates $4.3B in revenue for healthcare across platforms in 2024 with a CAGR of 24.6% between 2019 and 2024. [2]
Healthcare AI is Transforming the Industry from a Molecular, System, and Patient Level.
Molecular
AI applications in drug discovery have led to efficient and innovative methods for predicting protein structure and designing novel proteins. AlphaFold2, a machine learning application developed by DeepMind, can predict protein structures based on amino acid sequences alone with incredible accuracy making it particularly useful for identifying targets in drug discovery. It has predicted over 200 million structures to date. Its capabilities have now expanded to designing novel proteins, which some believe is the future of drug development. [3] Panelist, Morgan Cheatham, Vice President at Bessemer Venture Partners and medical student at Brown University, agrees and highlights these AI applications as biochemical breakthroughs at the molecular level of healthcare. The advent of this new technology will allow for drug developers to explore biochemical territory in new depths and at expedited paces.
System
On a systems level, rather than replacing physicians, AI can optimize the physician’s role by generating insights from large clinical data sets, triaging care, and leading to more accurate and earlier diagnoses. Panelist, Dr. Shrujal Baxi, Chief Medical Officer at Iterative Health and Oncologist, explains that in highly innovative fields like oncology it is not humanly possible to keep up with all of the clinical trials and novel therapies. With the use of AI, physicians can provide patients with the highest quality of care. Additionally, the hope is that with this AI application this high level of care can be achieved across all care settings and geographies.
AI has been used in radiology to improve triaging decisions and diagnoses. Aviceena’s AI CINA-iPE is a tool that analyzes CT scans for the presence of incidental pulmonary embolisms. This application allows for improved diagnostic capabilities and triaging decisions. [4] NIH’s Bridge to AI program is currently funding the development of an app that uses the sound of a patient’s voice to identify early predictors of Parkinson’s disease. [5] Additionally, an MIT-developed AI device is predicting early signs of Parkinson’s based on nocturnal breathing patterns. [6] Both AI developments could lead to earlier diagnoses and better outcomes for patients in one of the fastest-growing neurological diseases.
New atrial fibrillation detection devices use AI-guided ECGs to monitor and detect faulty heart rhythms before the onset of noticeable symptoms. [7] Further crediting AI with earlier detection of disease, which in turn will promote better outcomes for patients.
Patient
For patients, AI can lead to more timely care and improved outcomes. With AI-optimized physician roles, patients will experience a positive trickle-down effect. Cheatham states that, “Within the next 10 years, we will see a new world of ‘command-line medicine’ emerging. Where providers are not clicking boxes and sitting and waiting for lab results to come back, but rather they are using these models and methods to query this data with ease and to better understand the pathophysiology of diseases.” Dr. Baxi agrees and highlights that the role of the doctor needs to adapt for more optimal care. She says, “AI should help everyone get more palatable care by freeing up providers to do what they do best, but right now that is not the system.” Overall, thoughtful incorporation of AI into healthcare will improve the doctor's role and the outcomes for patients.
Consumers and clinicians worry that AI’s rapid adoption in healthcare could lead to significant consequences for ethics, patient privacy, and safety, but solutions are still needed.
Ethics
Algorithmic bias considerations are paramount in the successful adoption of AI in healthcare. While this has become a hot topic of conversation, companies have yet to find creative solutions to directly address this issue. According to Cheatham, Mayo Clinic is currently working on ways to investigate these obstacles, but there are still no clear answers in this area. Recent publications highlight the concern for largescale algorithmic bias without proper oversight and emphasize the need for developers to identify solutions. [8] In the near future, policy changes could result in developer consequences if proper actions are not taken to prevent algorithmic biases. [9] This further underscores the need for developers to incorporate these considerations into their models.
Patient Privacy
Although many AI datasets use desensitized patient information, many worry that well-trained algorithms will be able to re-identify these patients. In 2020, Mayo Clinic made the decision to share de-identified patient data to further AI innovation, but many are concerned that if this data is re-identified by strong algorithms that patients could face discrimination, social isolation, and job loss. [10]. While this concerned sentiment was shared by panelists, it was noted that many patients are not aware of how often and regularly they are sharing their data.
Safety
Patients and clinicians are concerned about the safety of AI, especially when historical examples have produced inaccurate or inconsistent outcomes. AI algorithms designed to identify skin malignancies concluded that rulers are malignant because diagnostic images with a ruler were more likely to be malignant. To avoid these outcomes, developers must pay close attention to inputs with variability or apply more stringent standards that may limit scalability. [11] This is a historical example that has led to more scrutiny of AI applications in healthcare. Cheatham explains that ‘model explainability’ is a critical consideration for the creation of safe and effective models in healthcare. When AI outputs cannot be explained, algorithms are no longer verifiably trustworthy. For example, ChatGPT, although trained on a massively large dataset, essentially the entire internet, is not always accurate in its outputs. [12] Although it may cite its source, that source does not always exist, and it may not be reliable. To mitigate this issue, critical assessment and intentionality in the type and size of datasets used to fuel algorithms in healthcare is crucial. Algorithmic care is based on clinical data, which is the same data used by clinicians to determine treatment and care plans for patients. The input is not changing, but the speed at which providers can assess this data could result in better more timely outcomes for patients overall.
Success of future AI in healthcare hinges on incorporating considerations for algorithmic bias, patient privacy, and safety into model development, collaborating with regulators and policymakers, and educating patients on AI use in healthcare.
With innovation, there comes a lag in necessary regulation and policy changes. It is critical for clinicians and AI companies to work with regulators like the FDA to agree upon adaptations. Dr. Shrujal Baxi explains that “We currently view privacy as a blunt endpoint, but many patients say that if their story could benefit even one other patient, they would gladly share it.” She highlights that incorporating these nuances into our understanding of privacy and asking why certain lines are drawn will be important to improve algorithmic care and patient outcomes. Dr. Baxi also emphasizes that “The FDA ultimately holds the keys to better patient outcomes and so in turn it is essential that we find ways to work together rather than view them as the enemy.” This sentiment was shared by Panelist, Lauren Koretzki, Senior Vice of Partnerships and Client Success at Wysa, Inc.
Finally, it is critical that patients are educated on how AI technology is being incorporated into their care. A recent survey found that 60% of adults are uncomfortable with AI in their healthcare but are not aware of how often it is used. [13] Adequate education and increased awareness of AI’s incorporation into healthcare will accelerate broader AI adoption in patient care.
References
- Hung, T. Performance of ChatGPT on USMLE: Potential for AI-assisted medical education using large language models. PLOS Digital Health. 2023. 2(2): e0000198.
- Global Data AI in Healthcare Report 2021.
- Eisenstein, M. AI-enhanced protein design makes proteins that have never existed. Nature Biotechnology. 2023. 1-3.
- Imaging Technology News. Avicenna AI Launches AI Solution for Incidental Pulmonary Embolism Detection. 2023. https://www.itnonline.com/content/avicennaai-launches-ai-solution-incidental-pulmonary-embolism-detection
- Artificial intelligence could soon diagnose illness based on the sound of your voice. 2022. https://www.npr.org/2022/10/10/1127181418/ai-app-voice-diagnose-disease
- Ouyang, A. Artificial intelligence model can detect Parkinson’s from breathing patterns. MIT News. 2022. https://news.mit.edu/2022/artificial-intelligence-can-detect-parkinsons-from-breathing-patterns-0822
- Mayo Clinic. Artificial Intelligence (AI) in Cardiovascular Medicine. 2023. https://www.mayoclinic.org/departments-centers/ai-cardiology/overview/ovc-20486648
- Panch, T. Artificial intelligence and algorithmic bias: Implications for health systems. J Glob Health. 2019. 9(2): 020318.
- Igoe, K. Algorithmic Bias in Health Care Exacerbates Social Inequities — How to Prevent It. Harvard School of Public Health. 2021. https://www.hsph.harvard.edu/ecpe/how-to-prevent-algorithmic-bias-in-health-care/
- Ross, C. At Mayo Clinic, sharing patient data with companies fuels AI innovation — and concerns about consent. STATNews. 2020. https://www.statnews.com/2020/06/03/mayo-clinic-patient-data-fuels-artificial-intelligence-consent-concerns/
- Narla, A. Automated Classification of Skin Lesions: From Pixels to Practice. J of Investigative Dermatology. 2018. 138, 2108-2110.
- Bogost, I. ChatGPT is Dumber Than You Think. The Atlantic. 2022. https://www.theatlantic.com/technology/archive/2022/12/chatgpt-openai-artificial-intelligence-writing-ethics/672386/
- Hagen, J. Survey: 60% of adults uncomfortable with AI in their healthcare. MobiHealthNews. 2023. https://www.mobihealthnews.com/news/survey-60-adults-uncomfortable-ai-their-healthcare?mkt_tok=NDIwLVlOQS0yOTIAAAGKM91N_2ChPrINqLCKmqh9aX-WdbZrL2PInuYhoVtnrfNLLMJbT--6J9aPxZ_MXXF1IztZVhDj1uhFbfoa7rZK_ZJo6xRSzVpVHkVNg4M-
###
Ashley Peake, Analyst and member of the metabolics and autoimmune practice at Health Advances.
Greg Chittim, Partner, Co-Leader of Health Advances’ Health IT and Digital Health practice.
Shomik Datta, Consultant and member of the oncology practice at Health Advances.